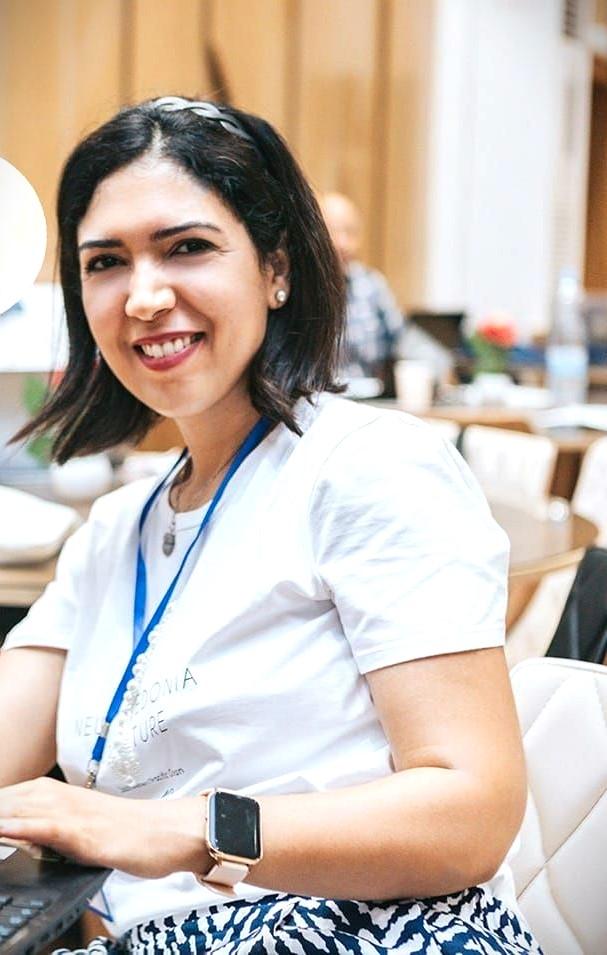
Lejmi Wafa
Establishment: US
Grade: Post-Doc
References | Publication | Link | |
---|---|---|---|
W. Lejmi, A. Ben Khalifa, M.A. Mahjoub, 2020, Traitement du Signal IF : 1.541, Q3, WOS, | 2020 | Link |
Searcher | Direction | Title | Country City | Presentation Date | Summary | Type | |
---|---|---|---|---|---|---|---|
Lejmi Wafa | M.A. Mahjoub, A. Ben Khalifa, | ISITCOM | 30 October 2021 | Review | Co-Encadrement |
Searcher | Direction | Title | Country City | Presentation Date | Summary | Type |
---|
References | Publication | |
---|---|---|
W. Lejmi, A. Ben Khalifa, M. A. Mahjoub, 2017, "Fusion Strategies for Recognition of Violence Actions", Hammamet, Tunisia, 30 October 2017 - 03 November 2017, Classée | 30 October 2017 - 03 November 2017 | |
W. Lejmi, M. A. Mahjoub, A. Ben Khlifa, 2017, "Event detection in video sequences: Challenges and perspectives", Guilin, China, 29-31 July 2017, Scopus | 29-31 July 2017 | |
W. Lejmi, A. Ben Khalifa, M.A. Mahjoub, 2019, "Challenges and Methods of Violence Detection in Surveillance Video: A Survey»,", Salerno, ITALY, 03-05 September 2019, Chapitre de livre | 03-05 September 2019 | |
W. Lejmi, A. Ben Khalifa, M.A. Mahjoub, 2022, "An Innovative Approach Towards Violence Recognition Based on Deep Belief Network", Istanbul, Turkey, 17-20 may 2022, Scopus | 17-20 may 2022 |
Projects | translate.Pôle de Recherche | |
---|---|---|
Analyse et traitement de documents (DAP) | ||
Intelligence Artificielle et Vision par Ordinateur (AICV) | ||
Intelligence Artificielle et Vision par Ordinateur (AICV) | ||
Intelligence Artificielle et Vision par Ordinateur (AICV) | ||
Intelligence Artificielle et Vision par Ordinateur (AICV) | ||
Intelligence Artificielle et Vision par Ordinateur (AICV) | ||
Intelligence Artificielle et Vision par Ordinateur (AICV) | ||
Intelligence Artificielle et Vision par Ordinateur (AICV) | ||
Analyse et traitement de documents (DAP) | ||
Analyse et traitement de documents (DAP) | ||
Analyse et traitement de documents (DAP) | ||
Analyse et traitement de documents (DAP) | ||
Analyse et traitement de documents (DAP) | ||
Analyse et traitement de documents (DAP) |
Cover page | Authors | Title | Link | ISBN | Publication date | Editions | Year |
---|
Project : DAP1: Word spotting
Word retrieval is an important task for understanding and exploiting document content by creating indexes. It is an information retrieval technique that aims to identify all occurrences of a query word in a set of documents (e.g., a book). In the word retrieval task, the input is a set of non-indexed documents and the output is a list of words ranked according to their similarity to the query word. This enables quick and easy online access to cultural heritage documents, and opens up further possibilities for studying these resources. In this project, we aim to improve word retrieval performance by developing a generative conditional model based on an adversarial network to generate clean document images from highly degraded images. This enhancement model deals with various degradation tasks such as watermarking and chemical degradation, with the aim of producing hyper-clean document images and fine detail retrieval performance.
The aim is to develop approaches for the identification/authentication of individuals using biometric measurements, based on new artificial intelligence (AI) and deep learning (DL) technologies. These approaches are relevant to applications such as securing access to protected infrastructures, and combating fraud, crime and terrorism.
Project : AICV 2: Intelligent embedded platform
The aim is to develop a platform for collecting/managing/storing digital data (images, audio, video) from public or private databases, under open or regulated license, or acquired from the sensors and cameras we have at our disposal. These data, which are essential to our studies, are constantly increasing in volume, on the order of Big Data, and changing in characteristics (size, resolution, modality), requiring a platform to facilitate their exploration/exploitation. It's a question of giving concrete form to the approaches developed in the form of hardware and software tools. These tools are the building blocks of embedded platforms and systems with real-time solutions for acquisition, analysis, object tracking, action recognition and decision-making.
When used in medicine, many augmented reality systems only consider a rigid registration of the preoperative model, and therefore do not take deformations into account. This reduces the credibility of augmented reality. In fact, the patient's position changes between the acquisition of the preoperative image and during the operation, when the table is often tilted to allow the abdominal viscera to slide to the bottom of the abdominal cavity to facilitate access to the organs. Heartbeats can also cause displacement. Also, the instruments will move and deform the organs, requiring new methods based on augmented reality to visualize these organ deformations during the operation. Various methods have been proposed to achieve satisfactory results, non-rigidly realigning the preoperative model with an intraoperative surface of the target organ. Unfortunately, these methods are unable to achieve good overall accuracy. The aim of this project is to explore new techniques.
Project : AICV 4: Intelligent transport systems
Although these technologies hold great promise for improving our current mobility habits and reducing the number of road accidents, there are still serious safety challenges to be addressed by Intelligent Transport Systems (ITS). In fact, although on-board intelligence in the automotive field has produced impressive results, particularly for environmental perception, these systems remain unable to overcome complex traffic situations. A real-time collaborative perception scheme in which vehicles no longer limit themselves to data acquired by their own on-board sensors, but exploit data from remote sensors, is needed for reliable perception of the environment. Vehicles are no longer seen as isolated systems controlled solely by their drivers, but rather as intelligent nodes distributed within a complex interconnected system. With vehicular wireless communication, ITS applications are supported by several high-performance communication technologies such as ITS-G5 and 5G. As a result, vehicles can interact actively and in a participative way using in-vehicle units and roadside units. In this project, we are targeting aspects such as pedestrian detection, multi-object detection and recognition of driver distraction actions.
The aim is to develop feature extraction approaches for the recognition of hand gestures with a jumping motion. Indeed, over the last few decades, hand gesture recognition (HGR) has become one of the most important research topics, due to its wide range of applications in computer vision. Various approaches and techniques have been suggested and evaluated to achieve significant results on HGR. However, even with the triumph achieved over state-of-the-art methods, they failed to take into account the non-linearity and non-stationarity existing on time series data, including raw Leap Motion Controller (LMC) data. In this research work, we propose a new method for extracting and selecting relevant and discriminating features using the Hilbert Huang transform (HHT) and Fisher discriminant analysis. Accordingly, CML time series signals are decomposed by an empirical mode decomposition. Next, HHT is applied to generate the resulting Hilbert marginal spectrum, and Fisher discriminant analysis is also performed for efficient feature selection.
The idea for this project is based on one observation: the imbalance between demand and production of electrical energy is growing exponentially, and available resources are being depleted at an alarming rate. At the same time, the customer's role remains passive in the face of this challenge. It is therefore necessary for the customer to participate in this system as a consumer-actor. The aim of this project is to develop predictive algorithms based on Artificial Intelligence and its application to the energy optimization of Smart Grids (SG).
This innovative research project focuses on signal reconstruction using new multi-wavelet-based approaches. By exploring advanced filters and algorithms, this research aims to improve the ability to reconstruct complex signals, with an emphasis on their application in the biomedical field. In particular, the study focuses on the analysis of electrocardiographic (ECG) signals, which are essential for the early detection of heart disease. In addition, the project extends to investigate signals associated with the coronavirus pandemic, notably in the context of medical data collected from COVID-19 patients. The expected results of this research will offer a deeper understanding of signal reconstruction, paving the way for better methods of detecting cardiac abnormalities, as well as providing essential insights into understanding variations in complex biomedical signals and their correlation with coronavirus cases.
Transcribing archival documents, particularly Arabic manuscripts, has remained a tedious and costly task for many years (often carried out manually by administrative staff or archivists). Three major contributions are included in this project. The first is a new method based on a deep UNet architecture adapted to identify the central part of each line of text. The second contribution concerns the proposal of a method based on a deep encoder-decoder architecture. The encoder consists mainly of five octave layers. The decoder consists of a succession of five layers of recurrent neural networks preceded by a layer integrating the deep self-attention mechanism. The third method is based on a deep encoder-decoder architecture, the encoder part of which is mainly based on the fusion of the skip connection technique and the gated mechanism.
The aim of this project is to extract information from visually rich documents (VRDs) using Graph Neural Networks (GNNs). RNGs were chosen because they excel at capturing relationships and dependencies between different components. This is particularly useful for DVRs, where components such as text units and encompassing frames have complex relationships. To this end, a first model has been proposed based on a node classification approach for extracting information from visually rich documents (VRDs) using a graph-based representation. The approach uses a weighted graph representation of VRDs, where node features are based on spatial, textural and visual characteristics extracted from the VRD, and node neighbors are chosen based on a customized edge weight. The document graph is then fed into a multi-layer graph convolutional network (GCN) for node classification, which is able to efficiently focus on important neighboring nodes.
Project : DAP 4: Opposing attacks
In this project, we propose to study adversarial attacks in situations relevant to real-world contexts by examining both sides of the issue: the ways in which an adversary can attack an implementation of a deep neural network, such as the sensors in an autonomous vehicle, and the ways in which these sensors can be hardened against adversarial attacks to ensure a reliable outcome. Recent implementations of machine learning-based applications tend to use multi-view and multi-modal data to accomplish their task, as single-view detectors show their limitations, particularly in the face of challenges such as occlusions. However, the majority of research on adversarial attacks focuses on single-view detectors, and adversarial attacks in a multi-view context remain a relatively understudied topic. One of the first aims of our work was to carry out an exploratory study into the transferability of patch-based adversarial attacks to different views of the same scene. This study showed that view angles have a significant effect on the performance of adversarial attacks, which has an impact on our objective: to propose an attack that can target a multi-view object detector. Initial findings show that for an adversarial attack to succeed, the attacker must simultaneously target multiple views. This multi-view adversarial attack will enable us to gain insight into the vulnerabilities specific to a multi-view context, leading us to our next objective: the implementation of an adversarial defense guaranteeing a highly robust deep neural network.
Atlas-based segmentation is a high-level segmentation technique that has become a standard paradigm for exploiting a priori knowledge in image segmentation. Different regions of the human body detected on medical imaging such as the brain or the female pelvic region, for example, are known to be anatomically complex and of high variability from patient to patient, making the task of segmentation using low-level segmentation techniques difficult. An atlas-based automatic segmentation approach using online learning has been developed. It was first applied to the segmentation of the human cerebellum from 2D brain MRI images. In a second step, the approach was applied to the segmentation of local regions likely to be affected by cervical cancer from 3D female pelvic MRI images. The proposed segmentation approach is based on a novel registration technique that uses a hybrid optimization procedure based on a particular genetic algorithm design combined with gradient descent in a multi-resolution strategy. The atlases used in this work were made available to us progressively in sequential order. The proposed approach is therefore based on an on-line machine learning method for the construction of the atlas base and for the segmentation process.
Project : DAP 6: 3D reconstruction of the thorax
The aim is to develop a digital model of the thorax, with the necessary meshing and simulation of the electrical impedance tomography (EIT) system on which image reconstruction is based. EIT scans the organ by passing an electrical current through the body, detecting it on the skin with an electrode belt and generating electrical impedance measurements. TIE is used to detect lung infections, breast cancer, etc., and is a non-invasive type of medical imaging. TIE for lung monitoring is based on the repeated measurement of surface voltages resulting from a rotating injection of high-frequency, low-intensity alternating current flowing between electrodes located around the chest. During monitoring, cyclic injections of electrical currents are performed sequentially, usually between all pairs of adjacent electrodes. Structural information from the human chest reflects the actual shape of the lungs and thorax. Several mesh sizes have been studied, leading to a specific number of nodes and elements in the mesh. The choice of the best mesh size was based on the smooth contour of the lung that was obtained. This method was used to study conductivity distribution within a section of the human thorax by varying the current injection pattern.
The main aim of the work carried out in this project is to implement a histological image analysis system capable of meeting all the constraints and difficulties of analyzing this type of large scene in the field of medicine. The approach, based on incremental phenotyping, will make it possible to analyze a WSI image and then obtain precise, targeted information. The system to be developed will support clinicians in the analysis and diagnosis of histological images. The system will assist medical experts in the morphological recognition of different cell types, enabling quantification and morphometric analysis (e.g. dimensions, circularity, texture, etc.) from digitized histopathology slides, with the aim of obtaining reliable quantitative data enabling correlation studies with the various issues raised by clinicians (diagnostic, prognostic and predictive). The aim of the project is to propose a system to assist clinicians in the analysis and diagnosis of histological images. This system will be integrated as an essential assistant in the automated professional workflows handled by clinicians.